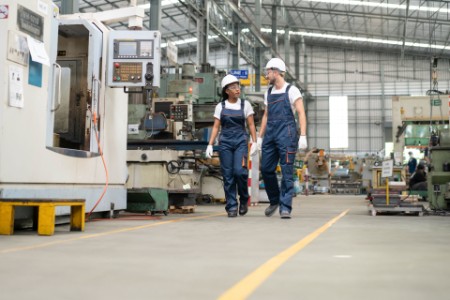
Chapter
Five initiatives industrial leaders can action to embed AI potential
Industrial companies can unlock real value with AI.
Despite uncertainty, industrial leaders can take steps today to ensure their companies are AI-ready. We have identified five cross-sector initiatives that address the challenges to AI deployment and differentiate between how companies can “act now” versus “evolve later.”
The pace of technological development calls for a two-speed approach to these initiatives. The “act now” recommendations require minimal investment, cause minimal disruption, are modular, and should show tangible results in the short term. As the wins compound and achieve momentum, “act now” recommendations grow into “evolve later” suggestions. These forward-looking suggestions require top-down strategic orchestration and capital allocation to create a sustainable flywheel for AI initiatives to grow.
These five key initiatives will help guide AI deployment towards being strategically aligned, operationally purposeful and commercially viable:
AI initiatives | Establish an AI value realization office and evolve into a control tower | Explore future scenarios to align the approach to AI | Develop a workforce reskilling plan | Create a data architecture assessment and upgrade roadmap | Develop AI ecosystem partnerships |
Act now | Set up an AI value realization office with a focused scope with C-suite accountability to test and learn | Use future-back planning linking AI to business value | Create a skills assessment to identify reskilling needs | Data architecture assessment | Map AI ecosystems and complementary capabilities and initiate pilots |
Evolve later | Scale up AI value realization office into a control tower | Continuous resource allocation to empower the company’s transformation across dimensions | Develop and implement a reskilling plan | Execute a data strategy with phased upgrades by ROI, impact and feasibility | Use key evaluation criteria to support a narrow but strong AI ecosystem of partners |
Initiative 1. Establish an “AI value realization office” and evolve into a control tower
Industrial companies should form a unit that streamlines experimentation and resources around AI and ties it to business outcomes. The value realization office coordinates knowledge sharing and shapes governance, but its primary goal is to realize benefits, conduct project and risk management, and optimize resources.
The value realization office can start with a smaller scope, but to extract maximum value from AI initiatives across the enterprise, it must evolve into a fully-fledged “control tower.” The control tower is a C-suite business unit, tasked with AI strategy and steering cross-organizational initiatives. It has the authority to allocate capital and coordinate resources across different business functions.
Act now: Set up an AI value realization office with a focused scope and C-suite accountability to test and learn
One way to experiment with AI is to create a value realization office via a simple project management office within a single business unit. This should involve stakeholders with different specializations, with C-suite support, focused on high-priority or quick-win projects, and actively analyzing and adapting governance practices to new business requirements. Leveraging easier-to-use technologies like GenAI and low-code or no-code can lower technical barriers to experimentation, enabling non-technical people to participate earlier. All of which increases buy-in, develops expertise, improves agility and enables activities that create value.
Evolve later: Scale up the value realization office into a control tower
As the value realization office grows in creditability and scope, companies can increase its autonomy and responsibility to scale up AI deployment with increasing C-suite oversight. It should evolve into a control tower, with formal positions, governance and resources to orchestrate projects companywide. The control tower would also orchestrate reskilling needs, data infrastructure upgrades and ecosystem strategies.
Initiative 2. Explore future scenarios to align the approach to AI
There is no shortage of potential use cases for AI, but organizations often struggle to align these use cases with overall strategy and vision.
By identifying scenarios for potential AI impacts and benefits, industrial companies can more efficiently allocate resources and prioritize initiatives.
Act now: Use future-back planning linking AI to business value
To develop focused initiatives aligned with an overall AI vision, industrial companies should begin with future-back planning, to identify the potential impact of AI on the business and the sector. Future scenarios should consider regulatory, macroeconomic, supply chain and resource constraints, and link AI activities to business value.
Evolve later: Allocate resources continuously to empower the company’s transformation across dimensions
To transition the value realization office into a control tower, link the top-down scenario planning and capital allocation with bottom-up learnings and activities. Scenarios can also inform which skills are needed for reskilling plans, data requirements for data architecture upgrades, and which competency white spaces exist for ecosystem strategies.
Initiative 3. Develop a workforce reskilling plan
AI is widely anticipated to have a sweeping impact on work and talent, as its rapidly advancing capabilities allow it to perform a wider variety of work with increasing ease and sophistication.
GenAI, in particular, can produce creative and analytical output like code, emails, images, slide decks — as well as taking over “blue collar” or “new collar” jobs in production. AI-enabled industrial robots are widening their roles by improving skills such as object recognition, learning and coordination, enabling them to play an increased role. GenAI could make factories more adaptive and efficient, by enhancing their ability to reduce unplanned downtime, enhancing forecasts.
The expanding role of AI will require workers to reskill, both to gain proficiency with using it and to enhance competencies that will become more valuable in the era of GenAI, such as quality assurance, content integration or customer engagement.
Act now: Create a skills assessment to identify reskilling needs
Begin by assessing what tasks AI is likely to take on and what competencies are required for workers, especially for blue collar work, where the impact of GenAI has been less explored.
Evolve later: Develop and implement a reskilling plan
Organizations should strive to create a continuous learning culture to enable them to adapt to constantly changing skills needs. Incentives can play an important role, linking reskilling to career advancement and financial compensation. And, by identifying and encouraging pockets of innovation, companies can keep talented employees engaged and attract new talent with in-demand skill sets.
Initiative 4. Create a data architecture assessment and upgrade roadmap
Industrial companies have traditionally deployed OT focused point solutions without a cohesive data strategy but having the right data architecture is critical for the effective deployment of AI across an organization. The challenge is compounded by the different kinds of data used by traditional AI (structured data) and GenAI (which excels at working with unstructured data). To use GenAI across the workforce, large language models (LLMs) should be trained on operating procedures and best practices — building a “knowledge graph” for the organization. But much of this information often resides only in the minds of employees, and may not be formally codified, much less stored in digital form.
Act now: Conduct a data architecture assessment
The data architecture should be evaluated to identify process design, dependencies, and data quality and security. Appropriate benchmarks can provide a performance baseline and support future AI business cases. Map the potential system upgrade scenarios by pairing phased upgrades with the potential ROI of corresponding use cases. Governance should be reviewed and strengthened to cover legacy risks (such as data privacy, bias and cybersecurity), as well as new risks created by new AI use cases.
Evolve later: Execute a data strategy with phased upgrades by ROI, impact and feasibility
After the infrastructure is mapped, companies need a strategy to collect, store and manage the data needed for AI applications. The first step is to identify and implement processes for boosting data quality. Secondly, they can adopt an ROI-driven, phased approach to capture data and introduce new use cases. Explore opportunities for new data acquisition, including “synthetic” data as needed to compensate for the lack of historical data for AI model testing and deployment.
Initiative 5: Develop AI ecosystem partnerships
Industrial companies are used to managing complex supply chain partner ecosystems. For both supply chain and AI partnerships, it’s important to vet partners, set performance standards and manage partnership costs.
However, AI partnerships bring greater complexity and depth of integration because AI solutions need to connect to central systems, be adaptable and managed over time. Each partner adds to the integration and management costs, impacting the orchestration of the technology stack. Underperforming AI partners can cause greater harm than traditional supply chain partners.
Act now: Map AI ecosystems and complementary capabilities and initiate pilots
When choosing partners for AI projects, companies should compare their AI capabilities, maturity and ecosystems against emerging best practices. Partners and ecosystems with complementary capabilities and experience can augment gaps in skills, technology and implementation. However, new partner and ecosystem relationships also require new governance. By establishing early partnerships with multiple entities, and identifying small pilot project opportunities, companies can build experience ahead of larger AI projects.
Evolve later: Use key evaluation criteria to support a narrow but strong AI ecosystem of partners
As the AI partner ecosystem evolves, it is essential to establish key criteria to evaluate the ecosystem relationships. This helps to select and develop relationships with priority partners and act decisively to remove unnecessary partners that fail to deliver value or adapt to future needs. As the ecosystem evolves, so should the function, governance and best practices playbook.
Impact of the five initiatives to address key challenges for industrial companies
Source: EY analysis, December 2023
Key considerations for industrial companies when deploying AI
Manufacturing companies have always been led by OT. AI won’t reverse that trend but will support a deeper IT/OT convergence. Business-driven point solutions are likely to grow in number and require the standardization and interoperability of OT data. Industrial companies should then recognize the need for a mature data architecture, to justify IT investments, and ensure AI brings value sustainably and safely. AI is enabled by technology but should be led by business.
Many of the benefits AI can bring to industrial companies come from the foundational work that needs to happen inside companies pre-deployment: It’s not solely a technology upgrade; it’s also an organizational and cultural upgrade.
The supply chain has existed for centuries and has always leveraged new technologies to adapt and improve. Today, supply chains that are more resilient, more cost-effective and more predictable are AI-enabled supply chains.
Some see AI as a buzz phrase, but it’s worth remembering that AI isn’t a switch, it’s a journey.
Special thanks to Francisco Almeida, EY Global Advanced Manufacturing & Mobility Analyst, Ernst & Young LLP; Michael S Fiske, EY Global Advanced Manufacturing & Mobility Analyst, Ernst & Young LLP; Anil Valsan, Director, EY Global Advanced Manufacturing & Mobility Lead Analyst, Ernst & Young LLP; Gautam Jaggi, Director, EY Knowledge Insights, Ernst & Young LLP and Claudio Knizek, EY-Parthenon Global Advanced Manufacturing & Mobility Leader for contributing to this article.
Summary
Industrial manufacturing companies are integrating AI into their operations to harness its transformative and disruptive potential. However, AI deployment also brings complex challenges, particularly regarding supply chain, strategy, people and IT.
Five initiatives can help guide companies toward more strategically aligned, operationally purposeful and commercially viable AI deployments. These initiatives include establishing a value realization office, aligning AI strategy with future scenarios, developing a workforce reskilling plan, creating a roadmap to upgrade data architecture, and curating AI ecosystem partnerships.