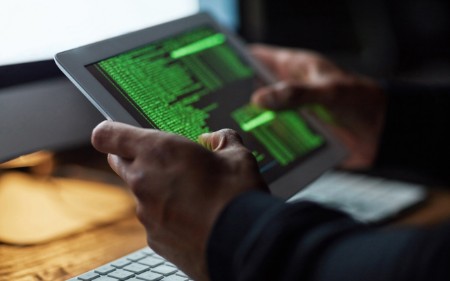
Chapter 1
The need and opportunity for change in AML
We need new approaches to combat money laundering.
At present, both the industry approach and regulatory framework are laboring in the fight against money laundering. Today, money laundering activity around the world is estimated to be between 2% to 5% of global GDP (pdf). Despite the vast resources deployed by financial institutions to combat money laundering, the current approach is not delivering results. According to a report by Europol, just around 10% of the suspicious transaction reports filed by financial services institutions lead to further investigation by competent authorities.
While financial services companies struggle to contain compliance costs, the industry can expect data volumes and the sophistication of threats to continue to increase. As a result, there is an increasing recognition of the need for innovation in AML and adoption of the latest technology.
The capability and impact of AI is growing rapidly
As AI capabilities have grown in recent years — demonstrated by real-life examples such as virtual assistants and robotics —its transformative potential has caught the imagination of businesses that are seeking to reduce cost, more effectively manage risk and increase productivity. Annual venture capital investment into US AI startups has increased by an estimated six fold since 2000 (pdf). AI’s potential to aid regulatory compliance has not escaped investors either. More than £238m of venture capital was invested in RegTech firms in the first quarter of 2017. Similarly, regulators and industry bodies such as the Financial Conduct Authority and the Financial Stability Board acknowledge the growing prevalence of AI in financial services and its applications to regulatory compliance.
Addressing the cost of compliance with AI
AI can drive significant efficiencies in typical operational hotspots, such as customer due diligence, screening and transaction monitoring controls. For example, incumbent AML transaction monitoring controls typically generate high levels of false positive alerts and significant operational workloads. The cost issue is further amplified by inefficiencies in the investigation process creating a significant divide between the efforts employed versus impact of transaction monitoring controls.
AI offers immediate opportunities to significantly reduce operational cost with no detriment to effectiveness by introducing machine learning techniques at different stages of the transaction monitoring process. AI is also being increasingly applied to customer due diligence and screening controls using natural language processing and text mining techniques.
Related article
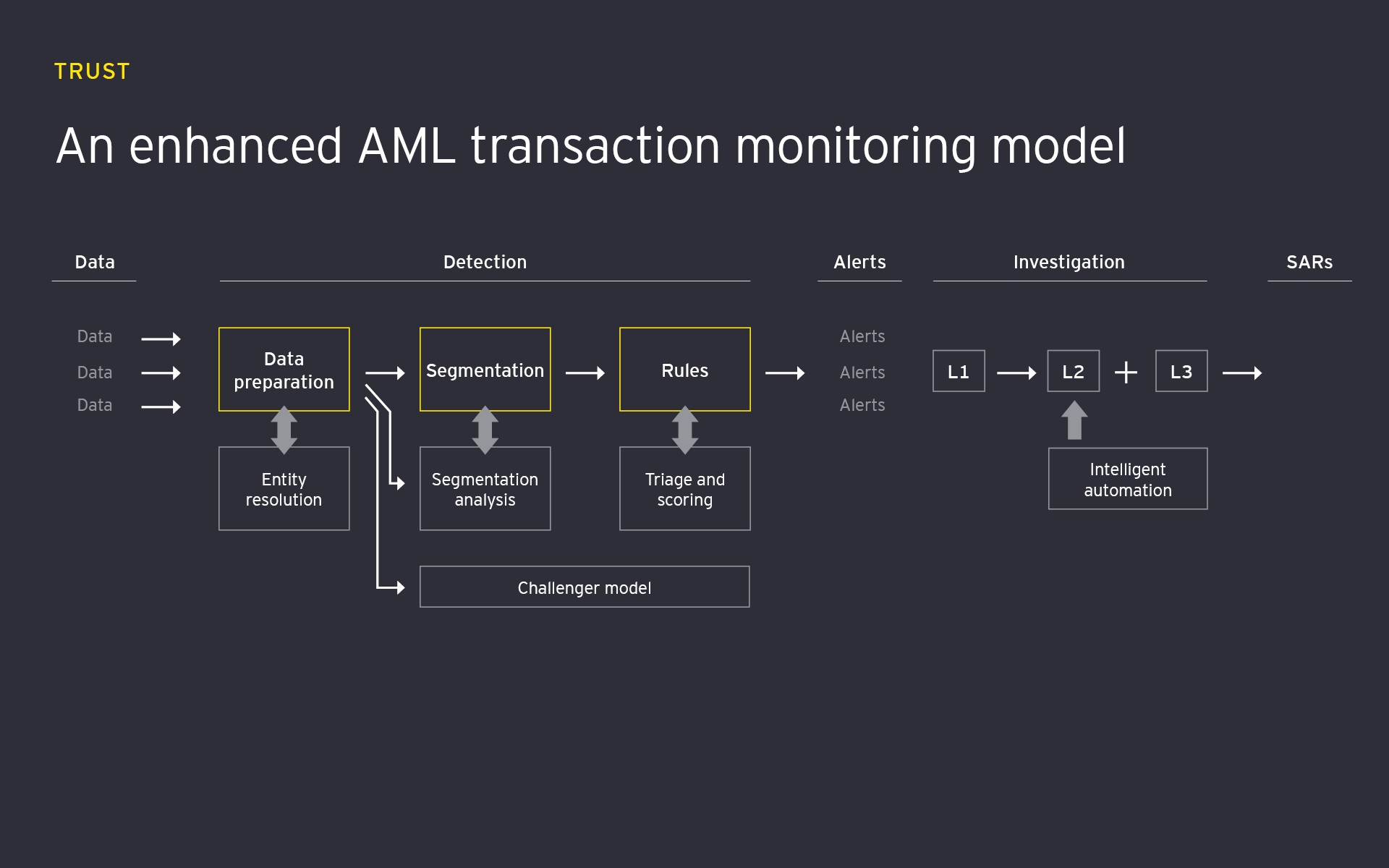
Integrated KYC — Bringing context back into AML with AI
The increasing understanding of how AI could be applied and integrated with human activity is driving new thinking in AML. It’s opening up opportunities that could lead to a fundamental shift in the approach to know your customer (KYC). Perhaps in the next wave of transformation we will see closer integration of risk assessment, monitoring, investigative and due diligence processes, with AI helping to break down silos and provide a more contextual basis for determining risk and detecting suspicious activity.
In this vision for the future, AI could bring increased breadth, scale and frequency to holistic KYC reviews in a way that better integrates ongoing screening and monitoring analysis. Risk and detection models would assess and learn from a richer set of inputs and produce outcomes in the context of both the customer’s profile and behavior. By leveraging AI’s dynamic learning capability coupled with skilled investigators, this model could be used to augment operations, provide quality control and even be used to train new resources.
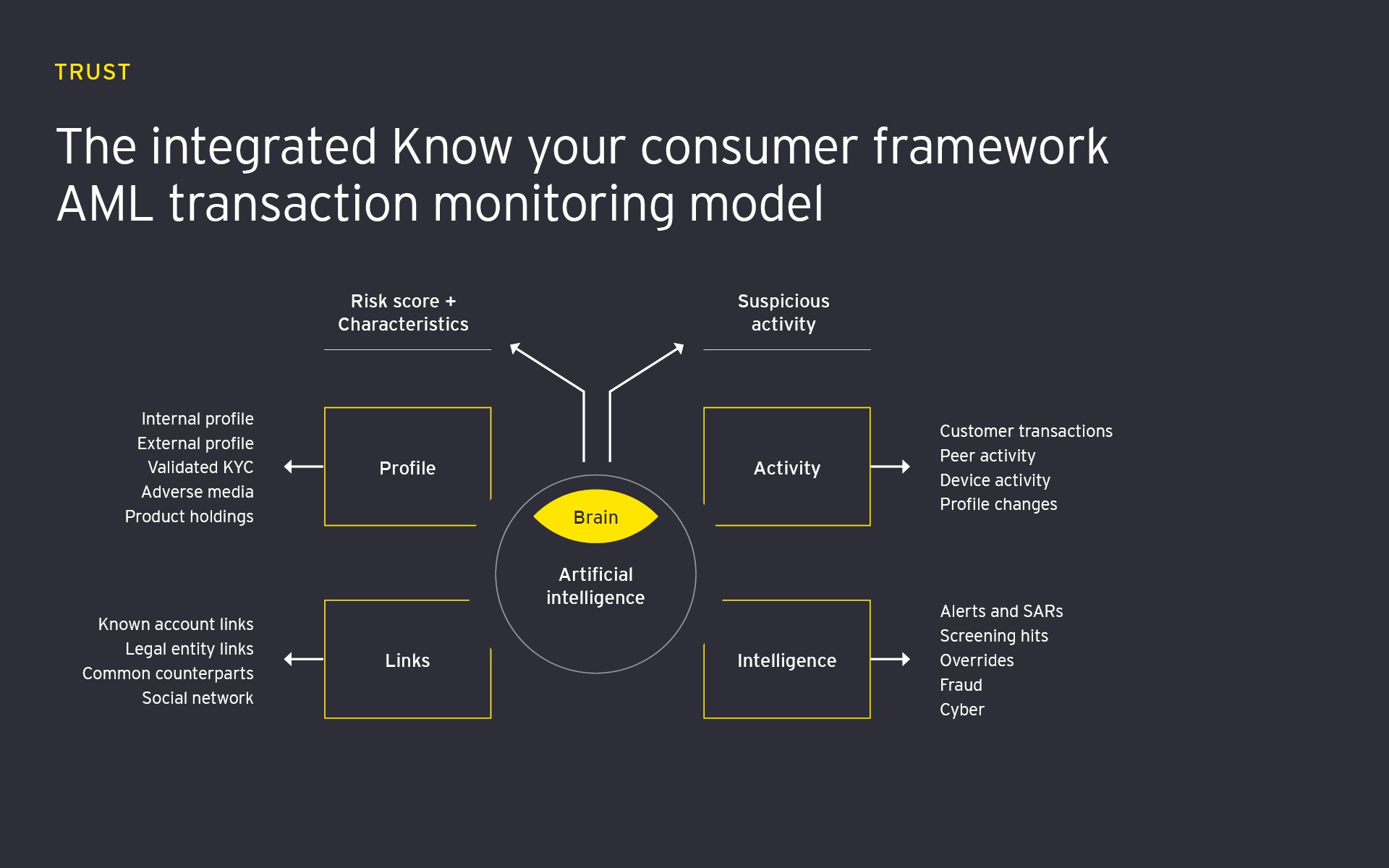
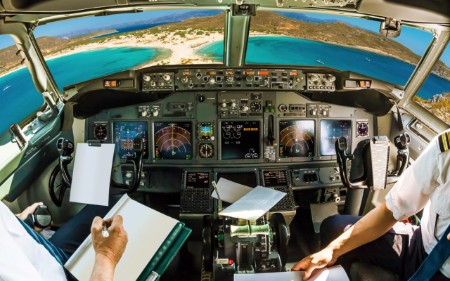
Chapter 2
How to build trust in AI applications
Seven ways to build trust in AI AML solutions.
Financial institutions are in a key position to explore opportunities and build trust in the AI applications for AML. Many organizations are, however, at the outset of their AI journey and hence may not be aware or well equipped to manage the new risks and challenges of deploying these new technologies. So what are the key dimensions of building trust in AI AML solutions?
1. Institute strong governance
Establishing strong governance and controls over the design, development and deployment of AI is critical to the safe and effective use in AML compliance. Good governance provides the means to assess and manage risk, promotes effective challenge and drives the necessary levels of understanding and documentation to inform effective decision-making across the life cycle of an AI solution.
A good starting point for developing AI governance and controls may be to leverage and adapt existing model risk management approaches (pdf) that have been increasingly applied to AML in recent years. Firms can use these and other similar regulatory pronouncements as a foundation from which to build a reasonable approach to AI adoption that addresses stakeholder expectations of risk management and oversight.
2. Define scope, objectives and success criteria at the outset
The basis for developing AI-enabled AML solutions must start with a clear statement of objectives to ensure that the design and implementation is aligned with the intended use and integrates effectively into business processes.
Defining what success looks like can be very difficult in AML as the outcomes and data sets can be highly subjective. Institutions may find that, without enhancing investigative and intelligence capabilities, AI will not yield significant benefits beyond incumbent controls. Establishing clear performance indicators and parameters, which link to a well-defined risk appetite statement, will be critical to tracking whether the outputs from the AI are meeting objectives at an acceptable level of risk.
Defining the scope of the AI solution comes next. Here, adherence to data protection policies, fair use of personal data and the legal right to explanation are an important considerations in deciding on the scope of data used to train and operate the AI as well as outputs and information that can be shared by the AI.
3. Make the design transparent
The ability to demonstrate and audit compliance is a cornerstone of the current AML framework — so the transparency of AI and its underlying algorithms is important. AI and machine learning is a broad field with varying levels of complexity and transparency. At the more complex end of the spectrum, neural networks and deep learning may prove more difficult areas in which to build trust, when compared with more existing processes. At present, very few of the current AML solutions being trialed in banks have advanced beyond regression, decision trees and clustering due to these challenges.
The design process has to consider AI’s different capabilities and appropriateness for the intended objectives and usage of the model, as well as the features of the input data. The design process should document the technical specification in detail along with known limitations and constraints of the proposed design for governance and stakeholder review.
4. Collaborate to define leading practice
Successfully embedding AI in the compliance ecosystem requires commitment and collaboration across multiple stakeholders: firms, vendors, regulators and government. Collaborative efforts can underpin wider adoption, and identification of further benefits, but also set standards for appropriate governance and controls to manage the safe development and deployment of AI-enabled solutions.
Greater adoption, collaboration and increased guidance can help drive forward AI innovation and deployment. Broader adoption, underpinned by regulatory convergence, will also help avoid asymmetries in control effectiveness that could that could otherwise push illicit activity away from more innovative institutions and further under the radar.
5. Focus on data inputs and ethical implications
The input data used to train and operate AI is critical. Data quality is a major challenge for many financial institutions and often impacts the effectiveness and efficiency of AML controls. Projects need to assess data quality and its appropriateness for use by AI as part of the design and development phase, and also implement data management controls to monitor the ongoing data quality during operation.
Another challenge with input data (particularly training data sets) is bias and the ethics of both the use of this capability and the nature of the trained AI. Recent high profile examples have highlighted the possibility of unintended consequences when trained on uncontrolled data inputs.
6. Apply robust testing and validation
The greater the level of testing and independent challenge the more effective the solution is likely to be and the less operational risk it will present. Common model risk management frameworks include model validation and independent model review teams that could provide effective challenge. Similarly, testing techniques such as stress and sensitivity testing as well as a champion/challenger approaches can be leveraged.
More novel techniques for validating AI applications could be drawn for other domains such as the use of red teams, bug bounties and secret shopper type approaches that are leveraged in testing and ongoing enhancements to cyber controls.
7. Engage early, deploy incrementally, review regularly
AI can bring significant disruption to compliance processes and institutions’ operating model. Engaging stakeholders early, building a common vision and deploying incrementally can help drive more effective change, constructive feedback and ultimately trust in business stakeholders.
When moving AI into production, organizations need to consider the operational risks that require ongoing monitoring controls. An increasing concern with promoting AI into everyday use is the possibility of malicious manipulation or unintended misuse. Periodic validation activities, including review of business use and sensitivity testing, can help mitigate risk along with regular review of AI decisions. At the same time, expert rules-based systems can be used to provide an ongoing baseline to compare to and help to identify where AI decisions deviate from expected norms.
Conclusion: It’s time to act
The current AML approach is struggling to keep pace with modern money laundering activity. There is a real opportunity for AI not only to drive efficiencies, but more importantly to identify new and creative ways to tackle money laundering.
While AI continues to pose challenges and test our appetite for risk, the question all financial institutions should be asking: can we afford not to embrace AI in our AML? Ultimately, when integrated with the right strategy and with the right focus on building trust, innovating with AI must be seen as a risk worth taking.
Summary
The question all financial institutions should be asking is: can we afford not to embrace AI in our AML?