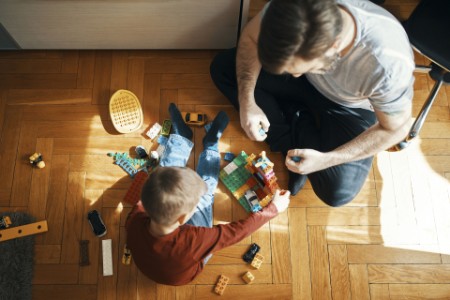
Chapter 1
The questions to ask
Organizations must adopt a holistic approach when trying to move up the data and analytics curve.
1. How will you manage change effectively?
Let’s start with the toughest challenge first. Change is difficult and to be successful organizations must establish a pull, rather than push, mechanism for adoption. Start off by communicating the vision and being transparent and honest about the objectives. Make sure people clearly understand the motivations behind the program and don’t start assuming the worst (such as trying to drop headcount with AI). Once the vision is public, pick a small group of willing participants and run a pilot. Finally, show the organization the results of the pilot – the ‘art of the possible’ to get people excited.
2. Do you have expert thought leaders to lead the change?
A chief analytics officer or a chief data officer can lend weight and experience to the journey toward data-driven decision making. Not only can they inspire, drive, educate and set the agenda to change both the tools and technologies in place, but also the thinking and culture of the organization. These leaders can enlist executive sponsorship, embed team champions, and have a voice at the table when whole-of-organization decisions are made. Traditionally, data has been an IT issue but today, leading organizations are recruiting and expecting a data-driven mindset in all of their senior leaders. Also consider keeping your data and analytics team small rather than employing too many deep capability experts. They may be perfect for today, but no longer right in 18 months. Work with external teams that complement your people while allowing you to scale up and down quickly as the need arises. You may have several alliances that deliver different skills for you and give you flexibility and options.
In tandem with leaders ‘walking the talk’ about the importance of improving data & analytics capability, it’s vital to have the right skills in your teams when you need them.
3. Do you see data as an asset?
To be truly successful, organizational leadership must agree that data is an asset worth investing in. From the boardroom to the C-suite, this focus on data and analytics driving decision making is crucial. To make this a reality, data quality and good data management will be required to embrace leading methods such as AI, neural networks and machine learning.
Never underestimate the importance of data assets; focus on building and maintaining solid data models, quality processes, and scalable frameworks.
4. How are you delivering value fast?
Design thinking, Agile methodology, Lean and Kanban are examples of rapid response approaches to help frame and address organizational challenges. Enhancing AI, data and analytics capabilities with these approaches can optimize business applications fast so that they keep pace with the speed of your teams.
Using rapid experimentation is a great way to show value fast. Allocate two weeks to a hypothesis and see what can be achieved. This will give a good sense of whether the data quality, technology and skills are there to deliver the desired result. It’s also important to clearly understand the different hypotheses, which ones are low risk and simple and which are hard with significant business risk. Balance the portfolio to ensure there is constant delivery while still pursuing the harder use cases. Make sure there is always value being delivered across basic business and simpler innovation cases while using the more complex transformational hypotheses to keep the business engaged in the process.
Set ambitious goals and let your teams drive the outcomes. They won’t all be achievable, but they will certainly focus your people and often, will yield some impressive outcomes.
5. Is your focus on today with an eye to tomorrow?
It goes without saying that working to an AI, data and analytics strategy linked to your enterprise strategy is a must. However, there is often disconnection between the corporate strategy and data and analytics. If the corporate strategy is the bright, guiding light, the data and analytics strategy is how you might achieve it. But it must be flexible, scalable and responsive to drive results. Develop the data and analytics strategy with a dual focus – some hypotheses that deliver to the needs of the organization today and others that look 12 to 18 months ahead. Test and experiment with the future-focused use cases and keep your technical architectures modular so you can add and change components as needed. Don’t lock into a single vendor solution which is the ‘old way’; embed flexibility to use emerging tech that will deliver the highest value.
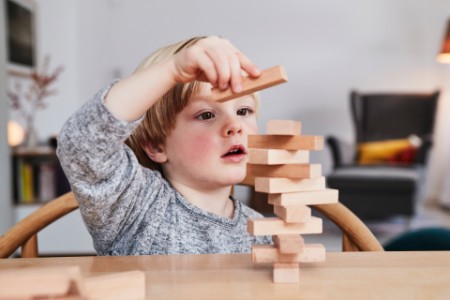
Chapter 2
Layered approaches help deliver value
Different approaches yield different results, it’s important to make the right choice for your organization.
Big bang
Some clients work to change their entire organization at once. They reposition themselves as digital organizations, rather than from their traditional industry. They dramatically change the structure of their teams such as giving authority for fast decision-making to small teams that may not necessarily be directly connected to the boardroom. It’s a disruptive approach and culturally challenging but becoming truly data-driven and analytics-enabled is potentially faster. We have one client that is repositioning from a traditional retail model in the financial services sector to a digital organization. The change is happening quickly and is challenging the business. This can be a difficult road to take.
Test and learn
A more common approach is to establish ‘speed to optimization’ methods and behaviors within targeted teams. For instance, organizations set up mini-capabilities which have the license to test and innovate. Anything successful is then handed onto another team to be embedded into the organization.
An example of this would be to take a traditional business intelligence team, flatten the structure and have them use agile sprints and rapid prototyping to deliver a minimum viable product (MVP). In our experience, organizations are definitely seeing immediate benefits from such teams.
It can be difficult to embed fast, ‘start-up’ style methodologies in large organizations, but we have seen it work successfully time and again.
Develop the right vendor alliances
The third approach involves working with vendor alliances that can help deliver value rapidly. If you find the right vendors, specialists in specific areas of data and/or analytics, they can help accelerate efforts and train your teams through ‘two-in-a-box’ approaches. Organizations collaborate to not only set the strategy, establish systems, infrastructure and processes, but to train up the in-house teams to sustain operations and add value in their own right. This approach is about both investing in a data and analytics capability and getting results quickly.
Any enterprise looking to improve their capability will find an approach that fits their needs. Keep a tight focus, learn, pivot and retest, act like a start-up, drive rapid results and celebrate the wins.
Think creatively about how you can rapidly progress along the data and analytics maturity curve. Look at how best to scale up your capability to get results fast.
Summary
Continual improvement through data modernization can feel overwhelming for businesses due to the sense that analytics technology is moving faster than they can keep up. In this article, we give insight into how businesses can continue to move up the data analytics maturity curve by adopting the right balance of current and future-state mindsets.