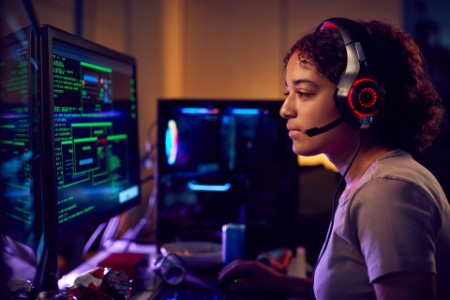
Chapter 1
Moving from preventive to predictive
For a long time, OEM guidelines were your best regimen to follow. But data offers a new way forward based on your operations and use cases.
No one questions the perfect day to repair or replace an asset: the day before your asset breaks down. But science hasn’t developed a crystal ball yet.
You’re likely following guidance from the original equipment manufacturer (OEM) about how to maintain your asset. But is that guidance intended to maximize the output and life span of your asset, or is it tailored to the OEM’s desire to make sure the warranties are kept up — or to prod you to invest more into the asset or buy a new one before it’s necessary? How was the asset tested to develop those guidelines — and how does that compare with how you’re actually using it?
Consider your car. You’re told to get an oil change after a certain number of miles or months. But what matters more than these metrics are the viscosity of the oil over time, its ability to handle heat, the length of your drives and how often you start the car. The most important factors aren’t continually monitored, so you’re left to follow imperfect thresholds that are up for debate — for instance, do you need an oil change after 3,000 miles or 3 months, or is it more like 10,000 miles or 6 months?
Preventive maintenance
89%of asset failures occur at random, not because the asset has reached a certain age, IBM research shows.
The answer is: well, it depends. Unless you have your own data on how the asset is performing and understand how it degrades, you have nothing else to go on but OEM guidelines. These guidelines are often flawed: IBM research shows that 30% of preventive maintenance activities occur more frequently than necessary, and 89% of asset failures occur at random, not because the asset has reached a certain age.
But now you can have the data; Internet of Things (IoT) sensors and other emerging technologies monitor how your assets are performing. You can move from preventive maintenance to predictive maintenance, according to predetermined conditions, instead of an often arbitrary schedule.
Predictive maintenance offers a 21st-century solution to a timeless concern among executives: how to get more from assets by reducing downtime and extending life cycles.
The power of predictive
IoT sensors on your asset produce data about factors such as pressure, vibration or viscosity. Artificial intelligence (AI) and machine learning monitor the data and trigger warnings when human intervention may be required. Using certain thresholds that you define, you can give yourself a cushion on maintenance depending on the risk level of failure and what the impact of that failure would be — whether it’s unplanned downtime at the more minor end of the spectrum or catastrophic damage on the other. The setup creates an automated way to schedule inspections and replacements, reducing or preventing asset failure while boosting equipment uptime.
IDC Manufacturing research shows that predictive maintenance plans can cut expenses up to 20% while boosting asset availability by 20%. Within the oil and gas industry, which is heavily reliant on expensive assets, IoT-enabled maintenance cut costs by 30% and equipment downtime by 45% and helped raise production output by 25%, the U.S. Department of Energy says.
“Predictive maintenance offers a 21st-century solution to a timeless concern among executives: how to get more from assets by reducing downtime and extending life cycles,” says Jim Perrine, Principal, Americas Enterprise Asset Management Leader, Ernst & Young LLP.
Aside from costing money and causing downtime, unneeded maintenance can backfire and deliver the opposite of what’s intended, reducing the life of your asset. Maintenance technicians cannot take apart an asset and put it back together as well as it was originally manufactured: every time they do so, a new failure point is potentially introduced.
Consider your home’s heating, ventilation and air conditioning system. Perhaps you pay a technician to clean and inspect your compressors every year. Every time you do a pressure test, you lose refrigerant, and, over time, you’ll eventually lose enough that you’ll then have to pay for more. But, with IoT-enabled sensors, such maintenance won’t need to occur until you’re notified that the refrigerant pressure is becoming too low.
There’s also an impact on safety, since some maintenance is potentially harmful to your workforce. In the manufacturing industry, 25% to 30% of workplace deaths are related to maintenance — due to shocks, burns and injuries from moving parts, for example — according to a report from BLR. Minimizing maintenance also means you’re not exposing your workers to unneeded risk.
And when you have the data, you’re set up for even more proactive possibilities. For instance, when you develop confidence in your AI capabilities, the technology can automatically start looking for replacement parts when needed and determine the availability from vendors if you don’t have them in stock.

Chapter 2
Rethink how maintenance is performed, not just when
Through digital and mobile enablement, your technicians can achieve more for less money, through smartphone apps or even AI image analytics.
When maintenance is required, digital enablement plays a role in getting the asset back to work as rapidly as possible, with an eye toward cost-effectiveness.
Skilled technicians generally must perform inspections and validations, in addition to completing the maintenance. These professionals are generally well compensated, and paying them to input that data into a system is wasteful, since it is not a task that requires deep knowledge. Yet, passing that data off to a less-skilled employee to input is also not efficient.
Digital enablement puts tools into their hands, like a smartphone app, without making their jobs more onerous and time-consuming. For example, voice-to-text functionality can eliminate laborious typing, and the need to scroll through multiple screens can be minimized.
Barcoding and scanning are also useful for collecting data from a reading on a machine. Say the reading on the machine is “103,” and your app has set a threshold that anything over 100 requires extra attention — the technician can be alerted while he or she is there performing the work, in real time. The reading isn’t handed off to an office worker who keys in a work order and schedules another visit, and the machine doesn’t need to be taken apart again.
The technician can also easily take a photo of the asset to document completion of the task, and, through pixel-to-pixel matching, trained AI can validate whether that asset looks proper after the maintenance.
You can also use a digital twin, a virtual representation of your asset and what role it plays in a process. Simulations based on performance data show when an asset may need work and when it could fail. You gain true insight into one piece of equipment and its components, with modeling that gets progressively more intelligent. Gartner has said that half of large industrial companies will gain a 10% boost in effectiveness by 2021 through using digital twins.
Putting standard solutions to work
The good news is that you don’t have to start from scratch to get these capabilities up and running. The software and hardware are on the market today, and you can get expert help in setting them up. The packaged software typically includes defined business processes intended to work across the board, regardless of sector or company size.
Companies can gain significant benefits by following these processes, yet some feel more inclined to bend the software to fit their existing processes through customization. Perhaps there’s a good reason for this, but the software will cost more to implement and require higher ongoing support costs. More importantly, these businesses won’t be able to tap into industry group benchmarking and effectively leverage data and optimization models honed over time, from similar assets, for analytics that drive better decision-making.
There’s a familiar adage: “If it ain’t broke, don’t fix it.” Through preventive maintenance, many companies are doing the opposite — and the outcomes can be measured in greater downtime, shortened asset life spans and ultimately lost revenue. Emerging technologies offer a different way forward — based on real-time data of your assets in the moment, not on schedules developed with no consideration of your unique goals, processes and use cases — that also enable smarter and safer ways of working and new predictive possibilities.
Jim Perrine, Principal, Americas Enterprise Asset Management Leader, Ernst & Young LLP, contributed to this article.
Summary
Using new maintenance models and certain condition thresholds, you can be more predictive and significantly drive down operational maintenance costs. IoT sensors on your asset produce data, and artificial intelligence and machine learning can monitor that data and trigger warnings when human intervention may be required, minimizing potentially disruptive and unnecessary maintenance. This approach allows you to maintain asset reliability using metrics that consider the risk level of failure and the impact of that failure, not just OEM guidelines.